Machine learning framework finds catalysts for lower-cost hydrogen production
Lauren Smith
Aug 22, 2024
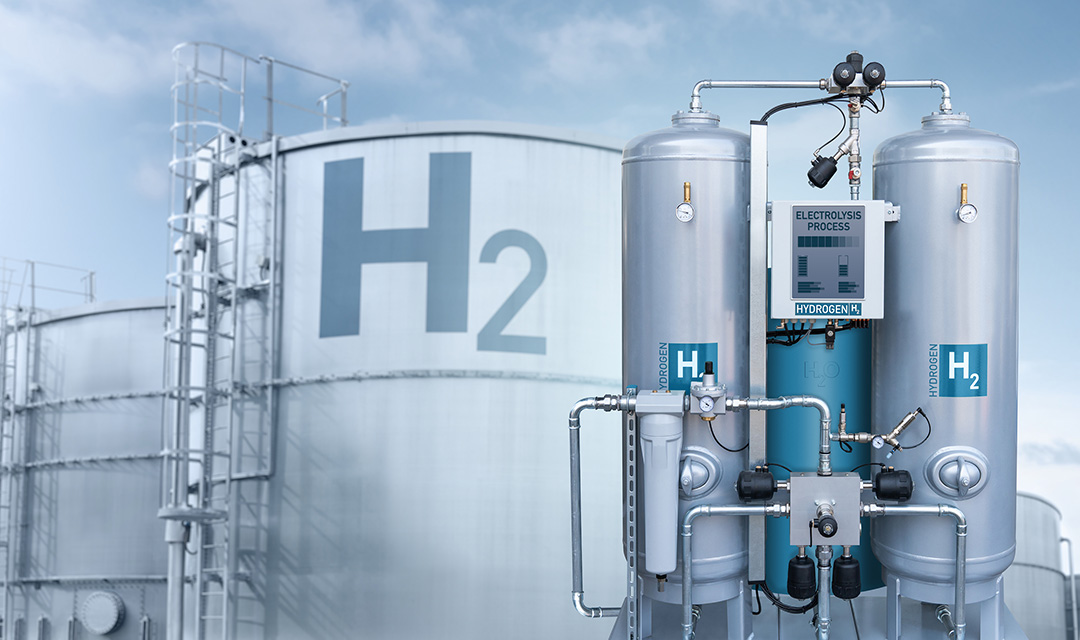
Until we have better catalysts, we are unable to meet the increasing demand for green hydrogen. Processes for generating renewable hydrogen often involve splitting water into hydrogen and oxygen. The state-of-the-art conditions for this reaction are acidic, causing many metals that would be used as catalysts to dissolve.
To identify a more stable catalyst, researchers at Carnegie Mellon University developed a machine learning (ML) framework.
Current water splitting processes rely on iridium oxide as the most active and stable catalyst. Iridium, classified as a critical mineral by the US government, is very expensive. To enable hydrogen production at lower cost and larger scale, we need to design efficient and stable alternatives.
Ruthenium offers one potential path. While it is more abundant and less expensive than iridium, it is also less stable and dissolves over time. Previous efforts in the field have focused on increasing the catalytic activity of ruthenium oxide. In the Journal of the American Chemical Society, Rohan Yuri Sanspeur, Javier Heras-Domingo, and John Kitchin bring more attention to its stability. Rather than rely on chemical intuition to identify potential alloys that would stabilize the ruthenium oxide catalyst, they developed an ML model.
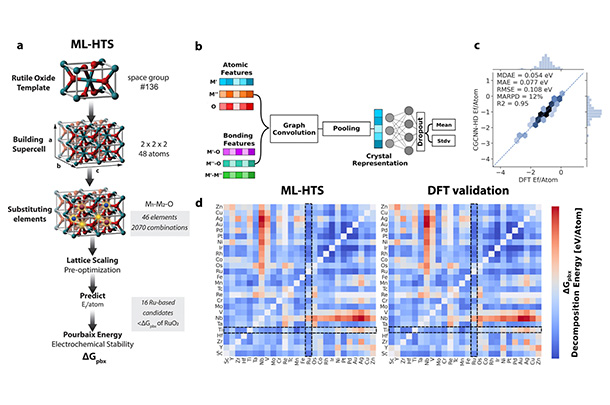
Source: J. Am. Chem. Soc. 2024, 146, 23, 15740–15750
a) Machine Learning (ML) High-Throughput Search (HTS) allows researchers to sift through the vast compositional and geometric space for acid-stable catalysts b) ML architecture used to map a crystal onto its acid-stability metric c) - d) Validation of the ML model against much more expensive first principles electronic structure calculations
Modeling the stability of a catalyst is hard. There are many possible aqueous forms that a catalyst might take under different reaction conditions. Existing databases are limited mainly to pure metals, and generating data for mixtures becomes a combinatorial challenge. "You can mix things in different ways, but it's not only a composition problem," explains Sanspeur, a Ph.D. student in the Department of Chemical Engineering. "For the same composition, you can have different arrangements of the atoms in space." The researchers used ML to address this challenge.
They trained their model on more than 36,000 mixed metal oxides, then used the model to predict the stability of hypothetical structures. "The ML-aided computational pipeline helped narrow the compositional space that we needed to search to find an improved catalyst," says Kitchin, a professor of chemical engineering.
The ML-aided computational pipeline helped narrow the compositional space that we needed to search to find an improved catalyst.
John Kitchin, Professor, Chemical Engineering
They found a candidate containing chromium and titanium. Collaborators at the University of Toronto synthesized the material and characterized it in different ways. Their experimental results confirmed it is more stable and more active than ruthenium oxide. The stability of the mixed metal composition over a thousand hour test is particularly significant because pure ruthenium oxide dissolves very fast.
To validate the activity of the composition, Sanspeur, Heras-Domingo, and Kitchin also used a semi-autonomous workflow they previously published with Zachary Ulissi. That work addresses the need for reproducibility in computational chemistry workflows. "We built a computational chemistry pipeline that tracks all your calculations, makes sure you know exactly what went into a calculation and when and how it ran. It's traceable and automated," says Sanspeur.
As he continues to build on the work, Sanspeur would like to develop a generative model that could allow the geometry and the space group of the material to change. The published ML model was only trained on the rutile phase of titanium dioxide. Rutile is a specific arrangement of atoms in space and is commonly used in experiments. "If we're no longer constrained to the rutile phase, we may be able to find an even better, more stable material," he says. Such a catalyst could make renewable hydrogen more economically feasible.
For media inquiries, please contact Lauren Smith at lsmith2@andrew.cmu.edu.